Your cart is currently empty!
AI and Ethics
Back to: MAIS AI Essentials
The Foundations of Ethical AI
Ethical Artificial Intelligence (AI) aims to align technology with human values, ensuring that its development and use benefit society. At its core, ethical AI prioritizes fairness, transparency, accountability, and respect for privacy. These principles are essential for building trust in AI systems and preventing unintended consequences.
Why Ethics in AI Matters
AI systems are increasingly integrated into critical areas like healthcare, finance, and education, influencing decisions that affect people’s lives. Without ethical guidelines, these systems risk perpetuating harm, such as reinforcing biases, invading privacy, or creating unfair advantages. Ethical AI seeks to mitigate these risks and ensure that technology serves the greater good.
Key Principles of Ethical AI

- Fairness: AI should treat all users equitably, avoiding discrimination based on race, gender, age, or other characteristics. This requires careful attention to the data used for training models to avoid replicating societal biases.
- Transparency: AI systems should operate in a way that is understandable to users. This includes providing clear explanations of how decisions are made and ensuring that users can challenge or appeal decisions when necessary.
- Accountability: Developers, organizations, and users of AI must take responsibility for its outcomes. This includes addressing unintended consequences and ensuring systems are designed with safeguards against misuse.
- Privacy: Respect for individual privacy is crucial. AI systems must protect sensitive data, obtain consent for its use, and avoid intrusive practices.
Ethical AI is not just a technical challenge; it is a societal imperative. By prioritizing fairness, transparency, accountability, and privacy, organizations can harness AI’s potential while minimizing harm and fostering public trust.
Bias and Fairness in AI
Artificial Intelligence systems are only as unbiased as the data they are trained on. When AI models rely on datasets that reflect societal inequities, they risk perpetuating or even amplifying those biases. Addressing bias and ensuring fairness in AI is essential for creating systems that treat all users equitably.
Understanding Bias in AI
Bias occurs when AI systems produce unfair outcomes, often because of patterns in the training data. These biases can take various forms:
- Historical Bias: Data reflects existing inequalities, such as wage disparities or underrepresentation of certain groups.
- Sampling Bias: Datasets may not represent the full diversity of the population, leading to skewed predictions or decisions.
- Algorithmic Bias: The design of an AI model may unintentionally favor certain outcomes, compounding existing disparities.
For example, an AI system used for hiring might favor male candidates if trained on historical data that reflects gender disparities in employment.
The Importance of Fairness
Fairness ensures that AI systems do not disadvantage individuals or groups based on characteristics like race, gender, or age. This is critical in applications with far-reaching consequences, such as:
- Healthcare: AI diagnosing tools must work equally well across different demographic groups.
- Lending: Credit risk assessment models should not unfairly deny loans based on racial or socioeconomic factors.
- Criminal Justice: Predictive policing systems must avoid disproportionately targeting specific communities.
Ensuring fairness is not just an ethical obligation—it is essential for maintaining public trust and ensuring the accuracy and reliability of AI systems.
Strategies for Mitigating Bias
- Diverse and Representative Data: Building datasets that reflect the diversity of the population reduces the risk of bias.
- Algorithmic Audits: Regularly testing models for unintended biases helps identify and address issues before deployment.
- Transparency: Clearly documenting the data and methods used to train AI systems ensures accountability and allows for public scrutiny.
- Inclusivity in Development: Involving diverse perspectives in the design and development process helps anticipate and mitigate potential biases.
By understanding and addressing bias, organizations can create AI systems that uphold fairness and equity, ensuring they work for everyone—not just a privileged few.
Privacy and Data Security in AI
Artificial Intelligence relies heavily on data, often including sensitive personal information, to function effectively. While this data-driven approach enables AI to deliver powerful insights and personalized services, it also raises significant ethical concerns about privacy and data security. Protecting user information is not just a technical challenge—it is a moral and legal imperative for ethical AI development.
The Importance of Privacy in AI
AI systems often require large datasets to learn and make predictions. This data can include sensitive information, such as:
- Personal Identifiable Information (PII): Names, addresses, social security numbers, and other unique identifiers.
- Behavioral Data: Online activity, purchasing habits, and preferences.
- Health and Financial Records: Medical histories, transaction logs, and credit scores.
Without proper safeguards, this data can be misused, leading to privacy violations, identity theft, or discrimination. For example, a data breach in a healthcare AI system could expose patients’ confidential medical records, causing significant harm.
Ethical Risks of Data Mismanagement
AI systems present several risks to privacy and data security, including:
- Data Collection Without Consent: Many AI systems collect data passively or through vague user agreements, leaving individuals unaware of how their information is being used.
- Mass Surveillance: Governments and organizations may use AI for intrusive surveillance, eroding personal freedoms and privacy.
- Data Misuse: Personal information collected for one purpose may be repurposed without consent, such as using fitness tracker data to determine health insurance premiums.
- Vulnerabilities to Cyberattacks: AI systems are not immune to hacking or breaches, which can expose sensitive information.
Data Security in AI
Data security focuses on protecting information from unauthorized access, ensuring its confidentiality, integrity, and availability. For AI systems, this involves:
- Encryption: Safeguarding data during transmission and storage to prevent unauthorized access.
- Anonymization: Removing or obfuscating identifiable information to protect individuals’ identities.
- Secure Access Controls: Limiting access to sensitive data based on user roles and permissions.
- Regular Audits: Monitoring systems for vulnerabilities and ensuring compliance with security protocols.
For example, an AI system used in banking should encrypt transaction data and implement multi-factor authentication to protect against fraud or theft.
The Role of Regulation

Laws and regulations play a critical role in ensuring privacy and security in AI. Key frameworks include:
- General Data Protection Regulation (GDPR): Mandates transparency and consent in data collection and provides users with the right to access, modify, or delete their data.
- California Consumer Privacy Act (CCPA): Grants consumers control over how their data is collected and shared, requiring businesses to disclose data usage practices.
- HIPAA: Protects health information in the United States, ensuring medical data is securely stored and accessed only by authorized individuals.
Organizations using AI must adhere to these regulations to avoid legal penalties and maintain public trust.
Strategies for Ethical Data Use
Developing AI systems that respect privacy requires thoughtful strategies, including:
- Data Minimization: Collect only the data necessary for the system’s purpose to reduce risks of misuse or breaches.
- Informed Consent: Ensure users are fully aware of how their data will be used, stored, and shared.
- AI Governance: Establish internal policies and oversight mechanisms to guide ethical data use and address potential risks.
- Privacy by Design: Integrate privacy considerations into the development process from the beginning, rather than treating it as an afterthought.
Balancing Innovation with Privacy
AI systems need data to function effectively, but this must not come at the expense of user privacy. Striking the right balance between innovation and ethical responsibility is crucial. For example, companies can use anonymized datasets to train AI models, preserving individual privacy while maintaining the model’s effectiveness.
Building Trust Through Transparency
Transparency is essential for fostering trust in AI systems. Users must be informed about:
- What data is being collected.
- How it is being used.
- Who has access to it.
Providing clear, accessible explanations builds public confidence in AI and encourages responsible data sharing.
Privacy as a Cornerstone of Ethical AI
As AI becomes increasingly embedded in our lives, protecting privacy and ensuring data security must remain top priorities. Ethical AI development goes beyond technical safeguards—it requires a commitment to respecting individuals’ rights and building systems that serve the public good. By adhering to these principles, organizations can harness AI’s potential while preserving the trust and safety of the people it impacts.
The Social Impact of AI
Artificial Intelligence is transforming society in profound ways, influencing how we work, communicate, and live. While AI offers numerous benefits, such as improving efficiency and driving innovation, it also raises challenges that require careful consideration to ensure equitable and ethical outcomes. Understanding AI’s social impact is essential to shaping a future where its advantages are shared widely and responsibly.
Transforming the Workforce
AI is changing the nature of work by automating tasks across industries. While this improves productivity and reduces costs, it also leads to job displacement, particularly in roles involving repetitive or manual tasks.
Opportunities Created by AI:
- New fields are emerging, such as AI ethics, data science, and machine learning engineering.
- Workers can focus on creative, strategic, or interpersonal tasks that AI cannot replicate.
Challenges of Automation:
- Low-skill and routine jobs are at the highest risk of being replaced by AI-powered systems.
- Reskilling and upskilling programs are essential to help workers transition to new roles in an AI-driven economy.
Governments, businesses, and educational institutions must collaborate to ensure the workforce adapts to these changes, balancing the benefits of automation with the need for employment opportunities.
AI and Social Inequalities
AI systems can exacerbate existing inequalities if not designed and implemented thoughtfully.
- Bias in AI Models: When trained on biased data, AI can reinforce societal prejudices, leading to unfair outcomes in areas like hiring, lending, or policing.
- Access to AI Technology: Wealthier individuals and countries often have better access to AI-powered tools, widening the gap between socio-economic groups.
To address these issues, developers must prioritize fairness and inclusivity in AI design, ensuring that the technology benefits diverse populations.
Privacy and Surveillance
The widespread adoption of AI has raised concerns about privacy and surveillance. AI systems often collect and analyze vast amounts of personal data, sometimes without users’ explicit consent.
- Mass Surveillance: Governments and organizations can use AI for monitoring public activity, potentially infringing on individual freedoms.
- Data Misuse: Companies may use collected data for purposes beyond the original intent, such as targeted advertising or price discrimination.
Balancing the benefits of AI-driven insights with the need to protect privacy requires robust regulations, ethical guidelines, and transparency in data usage.
Misinformation and Public Trust
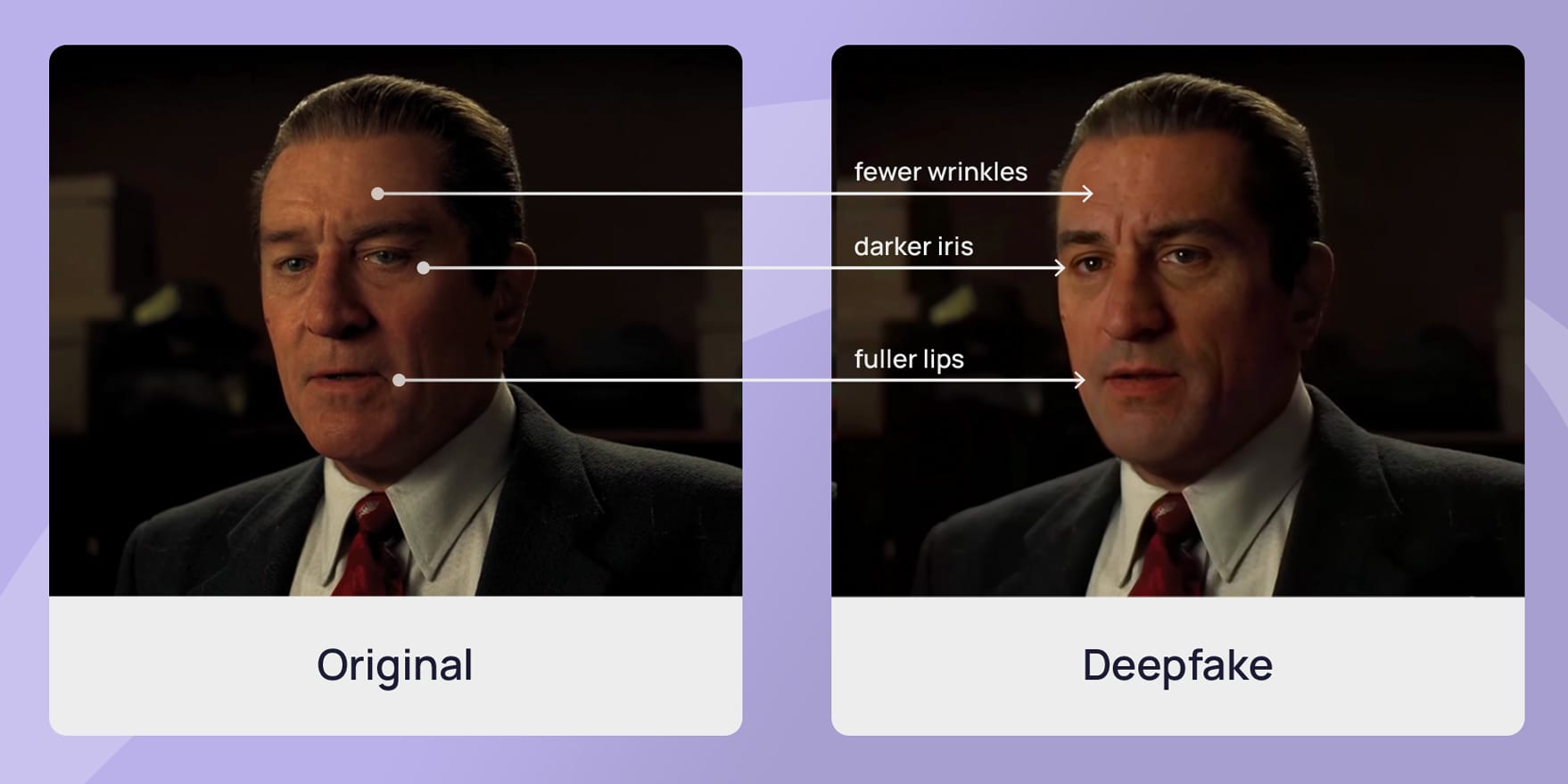
AI systems, particularly generative models, can create convincing fake content, including videos, images, and news articles. These technologies pose risks to public trust and democracy.
- Deepfakes: AI-generated media can spread misinformation or be used maliciously to impersonate individuals.
- Echo Chambers: Social media algorithms prioritize engagement, sometimes amplifying divisive or false content that aligns with users’ biases.
Addressing misinformation requires AI systems capable of detecting fake content, along with public education to improve digital literacy and critical thinking.
AI’s Role in Global Challenges
AI has the potential to address some of humanity’s most pressing issues, from climate change to public health.
- Sustainability: AI models optimize energy use, reduce waste, and support renewable energy adoption.
- Healthcare: AI aids in disease diagnosis, treatment planning, and resource allocation during pandemics.
- Education: AI-powered platforms provide personalized learning experiences, making education more accessible worldwide.
While these advancements are promising, they must be developed and deployed equitably to ensure that all communities benefit from AI’s capabilities.
Building a Responsible AI Future
The societal impact of AI depends on how we choose to develop and use the technology. Key strategies include:
- Inclusive Design: Involving diverse voices in AI development to address the needs and perspectives of all users.
- Ethical Frameworks: Establishing guidelines for responsible AI use, focusing on fairness, transparency, and accountability.
- Public Engagement: Educating the public about AI’s potential and risks to foster informed decision-making and trust.
By addressing these social implications, we can harness AI’s transformative power to create a more equitable, sustainable, and innovative future for everyone.
The Ethical Dilemmas of Self-Driving Cars
Self-driving cars, one of the most prominent applications of Artificial Intelligence, promise to revolutionize transportation by improving safety, reducing traffic, and increasing convenience. However, these vehicles also raise complex ethical questions about decision-making in critical situations. Understanding these dilemmas is key to designing AI systems that align with societal values and ethical principles.
The Role of AI in Autonomous Vehicles
Self-driving cars use AI systems to interpret their environment, make decisions, and control the vehicle. These systems rely on:
- Sensors: Cameras, radar, and LiDAR collect data about the car’s surroundings.
- Algorithms: Analyze the data to identify objects, predict their movements, and plan the car’s actions.
- Decision-Making Models: Choose the best course of action in real-time, such as stopping for a pedestrian or merging onto a highway.
While these technologies improve safety by minimizing human errors, they also introduce moral dilemmas when faced with unavoidable accidents.
The Trolley Problem: A Self-Driving Car Experiment
A classic ethical thought experiment, the “Trolley Problem,” is often used to illustrate the challenges faced by self-driving cars.
Scenario: A self-driving car is traveling down a road when it suddenly encounters an unavoidable situation. It must choose between:
- Swerving to avoid hitting a group of pedestrians but crashing into a wall, potentially harming the passenger.
- Continuing straight and hitting the pedestrians to protect the passenger.
This dilemma raises critical questions:
- Who Should Be Prioritized?: Should the AI prioritize the safety of the passenger or the pedestrians?
- What Factors Matter?: Should the AI consider the number of lives at stake, their ages, or their perceived value to society?
- Who Decides the Rules?: Should manufacturers, governments, or users determine how AI systems handle such decisions?
Ethical Considerations in Decision-Making
- Value Alignment: Ensuring the car’s decision-making aligns with societal and individual ethical values.
- Accountability: Determining who is responsible for the outcomes of the car’s actions—the developers, the manufacturers, or the passengers.
- Transparency: Making the car’s decision-making processes understandable to users and regulators.
These considerations highlight the need for clear guidelines and frameworks to govern the behavior of autonomous systems in morally complex situations.
How AI Can Mitigate Ethical Risks
While ethical dilemmas are inherent to self-driving cars, AI systems can be designed to minimize harm and prioritize safety:
- Risk Assessment: AI can calculate the least harmful outcome based on real-time data and preprogrammed ethical rules.
- Avoidance Algorithms: Advanced systems can reduce the likelihood of facing such dilemmas by predicting and avoiding dangerous scenarios earlier.
- Human Override: Autonomous vehicles can include options for passengers to take control in extreme situations, ensuring ultimate accountability remains with humans.
The Future of Ethical Self-Driving Cars
As self-driving cars become more common, addressing these ethical questions is essential to building public trust. Governments, manufacturers, and ethicists must collaborate to develop standards that ensure these systems operate responsibly.
Self-driving cars are a glimpse into the broader challenges of AI ethics: balancing innovation with moral responsibility. By tackling these dilemmas head-on, we can create AI systems that reflect our values and prioritize the well-being of all individuals.
Copyright 2024 Mascatello Arts, LLC All Rights Reserved