Your cart is currently empty!
AI and Self-Driving Cars
Back to: MAIS AI Essentials
The realm of artificial intelligence (AI) has ushered in a new era of possibilities, revolutionizing various sectors, including transportation. One of the most captivating applications of AI is its integration into self-driving cars, also known as autonomous vehicles.
Overview of Self-Driving Cars
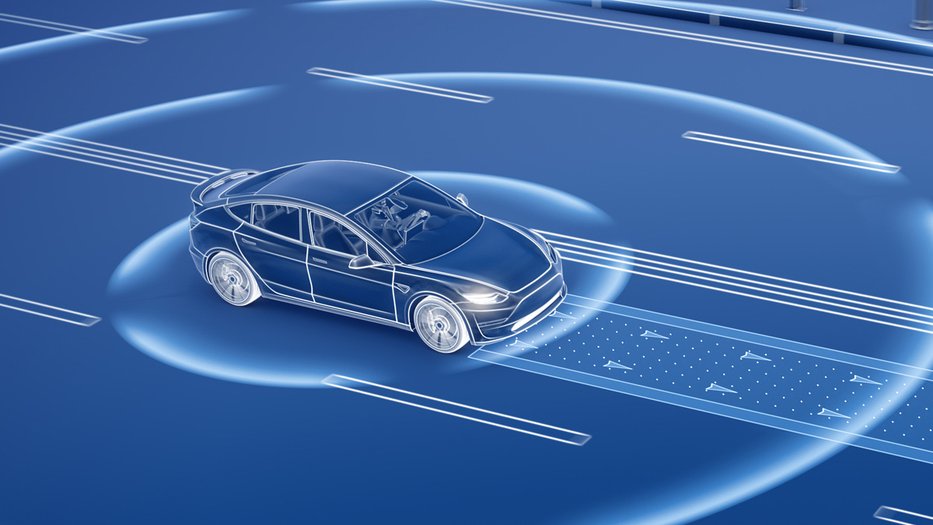
Photo Credit: CalTech Science Exchange
Imagine a car that drives itself! That’s an autonomous vehicle (AV), also called a self-driving car (SDC). A combination of technologies senses the surroundings and navigates without a human at the wheel. Just as we perceive the world with eyes and ears, and use a map or GPS to find our way, the major parts that will make AVs work are:
- Sensors: These are like eyes and ears for the car. Cameras, radar, and LiDAR (like radar, but with lasers) work together to create a 3D picture of the road and everything around it.
- Artificial Intelligence (AI): This is the brain of the AV. It receives information from the sensors and uses complex algorithms to decide what the car should do next, like accelerate, brake, or turn.
- Software: Think of this as the instructions for the AI. It tells the car how to interpret the sensor data and make safe driving decisions and determine how to find the way to its destination.
There are different levels of autonomy, for example”, 1) needing a human driver ready to take over (like some advanced driver assistance systems), or 2) a fully self-driving car (still under development), and 3) several levels in between.
Here is a list of software that is present is today’s Tesla’s

Tesla vehicles are equipped with advanced software that covers a wide range of functionalities, from infotainment to autonomous driving. Here are the key types of software that Tesla uses:
- Autopilot and Full-Self Driving (FSD) Software: Tesla’s semi-autonomous system with lane-keeping, adaptive cruise control, and automatic lane changes, powered by cameras, radar, and machine learning. An advanced version of Autopilot designed for autonomous driving, handling tasks like freeway merging and recognizing traffic signals. Continually updated via over-the-air (OTA) updates, with neural networks driving real-time object detection, path planning, and decision-making.
- Infotainment System: Tesla’s custom operating system powers entertainment, navigation, and vehicle controls. Navigation is based on Google Maps, integrating real-time traffic and Supercharger locations. Media services like Spotify, YouTube, and Netflix are available, with voice commands for controlling media, navigation, and settings.
- Over-the-Air (OTA) Updates: Tesla frequently delivers OTA software updates to enhance features, improve functionality, and fix bugs. These updates can enhance areas like battery efficiency and Autopilot performance, allowing Tesla vehicles to improve over time.
- Energy Management Software: Tesla optimizes battery use and charging through software that manages regenerative braking, Supercharging rates, and real-time energy consumption feedback.
- Mobile App Integration: The Tesla mobile app allows remote control of the car, including unlocking, starting climate control, and monitoring charging. It integrates with the car’s navigation for route planning and locating charging stations.
- Security Software: Sentry Mode monitors the car’s surroundings when parked, using cameras and sensors to detect suspicious activity and notify the owner via the app. The built-in dashcam records video footage using the car’s cameras.
- Vehicle Controls and Diagnostics: Software controls all interior features like climate control, heated seats, and “dog mode” for pets. Real-time diagnostics monitor vehicle health, allowing Tesla to remotely detect and sometimes fix issues without a service center visit.
- Artificial Intelligence and Machine Learning: Tesla uses AI and machine learning extensively, especially for self-driving capabilities, training its models with data collected from the entire fleet of Tesla cars.
Tesla vehicles are essentially rolling computers, constantly connected to Tesla’s cloud, where data is analyzed and new features are pushed through software updates. This integration of cutting-edge software and AI gives Tesla vehicles many of their unique capabilities.
Why are AV’s interesting?
- Safety: AVs could potentially reduce traffic accidents caused by human error.
- Convenience: Imagine getting in a car and relaxing while it drives you to your destination.
- Accessibility: Self-driving cars could provide transportation for people who can’t drive themselves.
The Challenges with AV’s:
- Technology: AV’s need to be incredibly reliable and handle unexpected situations.
- Laws and Regulations: There are still many legal questions about how AVs will operate on roads.
- Ethics: Who is responsible if an AV crashes?
History of Self-Driving Cars
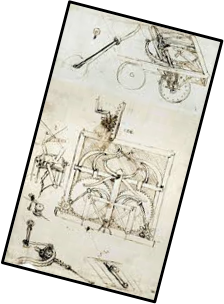
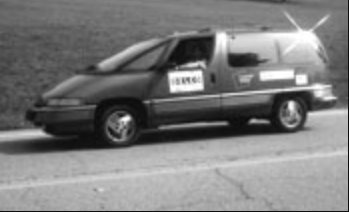
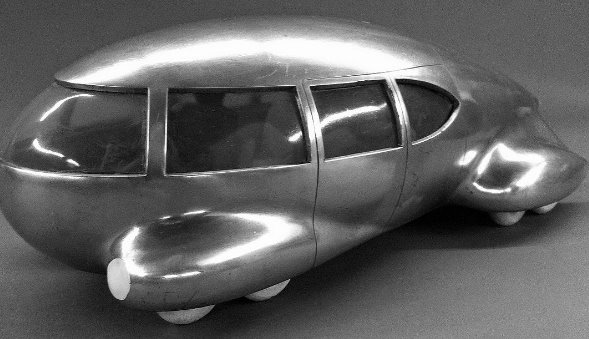
The idea of a self-driving car is surprisingly old:
- Early Dreams (16th Century): Leonardo da Vinci sketched a self-propelled
cart with a pre-programmed steering system, the first concept of an autonomous vehicle. - The “Show Car” (1939): Norman Bel Geddes designed a self-driving
electric vehicle for a GM exhibit. It used radio signals and magnets
in the road for guidance. - Test Tracks (1950s & 60s): Researchers in the US and UK experimented
with self-driving cars on controlled tracks, some using cameras and
magnetic cables for guidance. - The First Autonomous Cars (1980s): Carnegie Mellon University and
Mercedes-Benz independently developed self-navigating vans using
cameras and early computers. - The 1990s, Carnegie Mellon’s Navlab 5 successfully completed
a cross-country trip from Pittsburgh to San Diego, demonstrating
the potential for long-distance autonomy. - DARPA (Defense Advanced Research Projects Agency): research led to internet, and self-driving military technology.
- The 2000s: Interest from major corporations like Google (Waymo) and automakers like Toyota and Audi, increasing the power of sensor technology and artificial intelligence. Computer power fueled faster development and systems. Tesla raised the bar higher on electronic vehicles EV, and their own “assisted driving” strategy.
- The 2000s: Interest from major corporations like Google (Waymo) and automakers like Toyota and Audi, increasing the power of sensor technology and artificial intelligence. Computer power fueled faster development and systems. Tesla raised the bar higher on electronic vehicles EV, and their own “assisted driving” strategy.
Since then, the idea has kept evolving:

- Advanced Sensors: LiDAR and improved cameras provide a much clearer picture of the environment.
- More Powerful AI: Very powerful processors can handle complex calculations for data interpretation and better decision-making on the road.
- Focus on Safety: Redundant systems and safety protocols are being developed to minimize risks.
There’s no single inventor or invention, but rather a long history of contributions that have shaped the idea of the self-driving car. Today, the focus is on developing reliable and safe technology to make this futuristic concept a reality on the roads and highways.
Benefits of Self-Driving Cars to Society
Impacts
Self-driving cars will impact daily life and how cities are planned in the future.
For Everybody
- Commute Time Reimagined: All those hours spent stuck in traffic! Self-driving cars free up your time to work, relax, or catch up on a book while the car does the driving.
- Increased Mobility: No driver’s license? No problem! AVs could offer safe on-demand transportation for those unable to drive due to age, disability, or other reasons.
- Enhanced Safety: Human error is causes most of accidents. AVs relying on advance technology will reduce crashes making roads safer for everyone.
- Reduced Stress: Goodbye to white-knuckle commutes and road rage! AVs handle stressful situations like heavy traffic or bad weather, and you arrive at the office feeling calm and collected. Better yet. Arrive at the beach and hit the sand, chill out.
- More Productivity: Or maybe the CAR IS your office! With a self-driving car, you use your travel time for emails, calls, or even video conferencing.
Planning for the Future:
- Smarter Cities: Imagine cities designed around traffic
flow, not just car storage. AVs will optimize road use,
reducing congestion and allowing for more
pedestrian-friendly areas and public spaces. - Reduced Emissions: Smoother traffic flow and more fuel-efficient AV technology
may lead to a significant decrease in vehicle emissions, benefiting public health. - Improved Public Transportation: Self-driving cars will integrate seamlessly with existing public transportation systems, offering first-and-last-mile solutions
making it easier to get around without a car. - Economic Opportunities: The development and use of AVs will create new jobs in
areas like maintenance, programming, and infrastructure management. - Increased Accessibility: On-demand self-driving car services or subscriptions could provide reliable and affordable transportation options in areas with limited public transport or for people with special needs.
There are challenges for these benefits to be a reality, ensuring the safety and reliability of the technology and developing clear regulations for their operation. Self-driving cars will improve our daily lives and shape the future of transportation.
Impacts

Social, Environment, and Cultural Impact: The ubiquity of self-driving cars has the potential to bring about significant changes in society, culture, and the environment. Some of the impacts:
Society
- Increased Safety: Self-driving cars might drastically reduce traffic accidents caused by human error, making roads safer for everyone.
- Improved Accessibility: People unable to drive may gain more independence with self-driving cars.
- Transformation of Jobs: Professional drivers (taxi drivers, truck drivers, etc.) jobs might decrease, leading to displacement in this sector.
- Shift in Car Ownership: Car ownership would decrease. Ride-hailing services or subscriptions would be accessible and affordable.
- Changes in Urban Planning: With less need for parking, cities redesign streets to prioritize pedestrians, cyclists, and green spaces.
Environment
- Reduced Emissions: Likely electric self-drives make for fuel efficiency and lead to reduced greenhouse gas emissions and air pollution in urban areas.
- Traffic Congestion: Self-driving cars communicate with each other and infrastructure, leading to optimized traffic flow and reduced congestion.
- Increased Energy Use: Wider adoption of self-driving cars, could lead to a net increase in energy consumption.
- Rethinking Urban Sprawl: The convenience of self-driving cars could lead to living further from work and amenities, increasing urban sprawl.
Culture
- Redefinition of Freedom and Control: Self-driving cars may change our relationship with cars. The idea of leisurely driving might fade.
- Increased Productivity and Leisure Time: Time spent commuting could be used for work, entertainment, or relaxation.
- Potential for Social Isolation: Less interaction with others who drive could lead to more social isolation.
Technology of Self Driving Cars
Dramatic advances in electronics, computing and automation have brought us to where we are today. An Autonomous Vehicle describes something that can operate independently, with no constant outside control.
1. Self-Governing: An autonomous car has its own set of rules or internal mechanisms that guide its actions. This could be a pre-programmed set of instructions or the ability to learn and adapt based on its environment.
2. Limited External Control: While some external oversight might be present, an autonomous car doesn’t require constant human intervention to operate. It can make its own decisions within its defined parameters.
3. Levels of Autonomy: Autonomy is specified on a scale (Level 0-5). Self-driving cars, for instance, might require some human intervention in complex situations, while a thermostat might operate with very little outside influence.
Examples of Autonomous Systems:
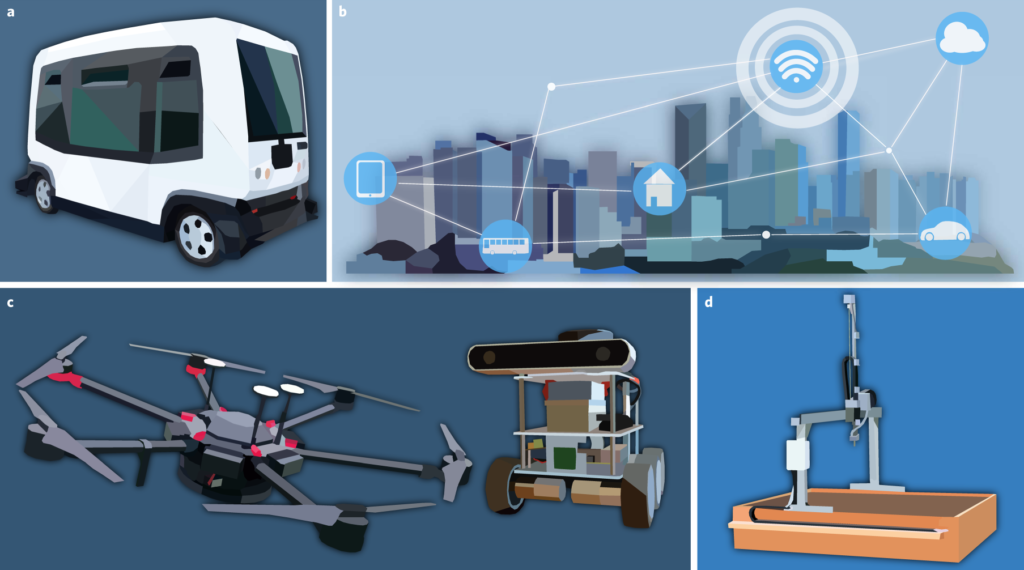
Photo Credit: nature&ecology evolution
- Robots: Industrial robots can perform complex tasks with minimal human input
- Self-driving cars: These vehicles use sensors and Artificial INtelligence to navigate roads without a driver.
- Drones: These unmanned aerial vehicles can fly pre-programmed routes or respond to real-time instructions.
- Thermostats: They automatically adjust room temperature based on a set point.
Society of Automotive Engineers (SAE)
The Society of Automotive Engineers (SAE) provides a standard, “Recommended Practice: Taxonomy and Definitions for Terms Related to Driving Automation Systems for On-Road Motor Vehicles”, commonly referenced as the SAE Levels of Driving Automation™, the industry’s most-cited source for six levels of driving automation. Place holder – TBD
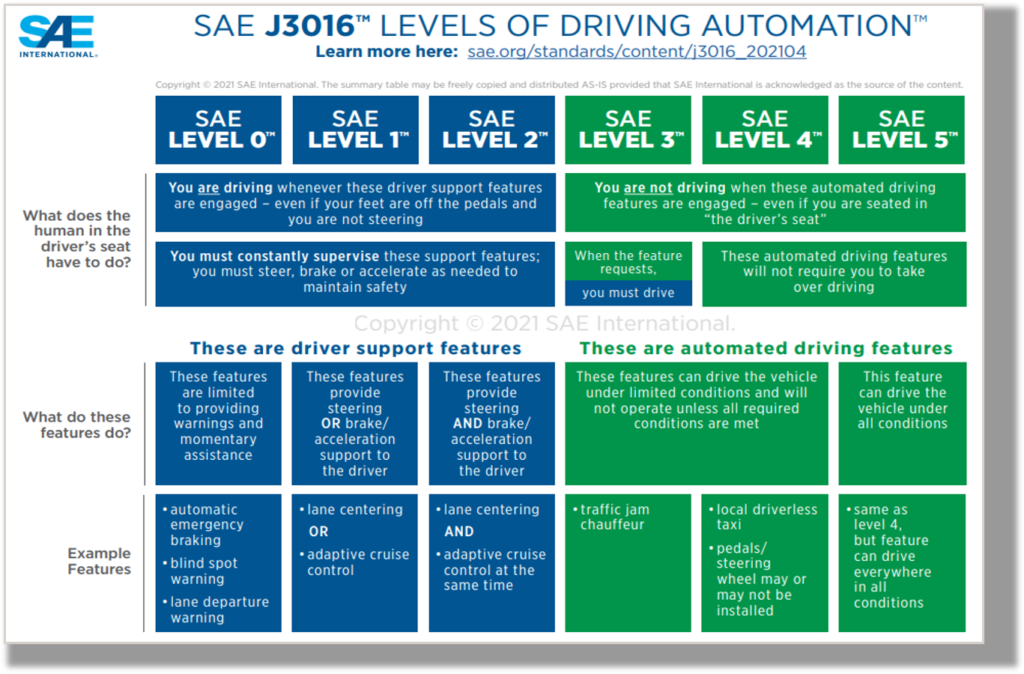
This table provides a quick overview of sensor or sensor-like systems for the car to determine its location, perceive the environment, spaces, and obstacles around the car, and move the car safely along the road. The following slides provide more detail about these technologies.
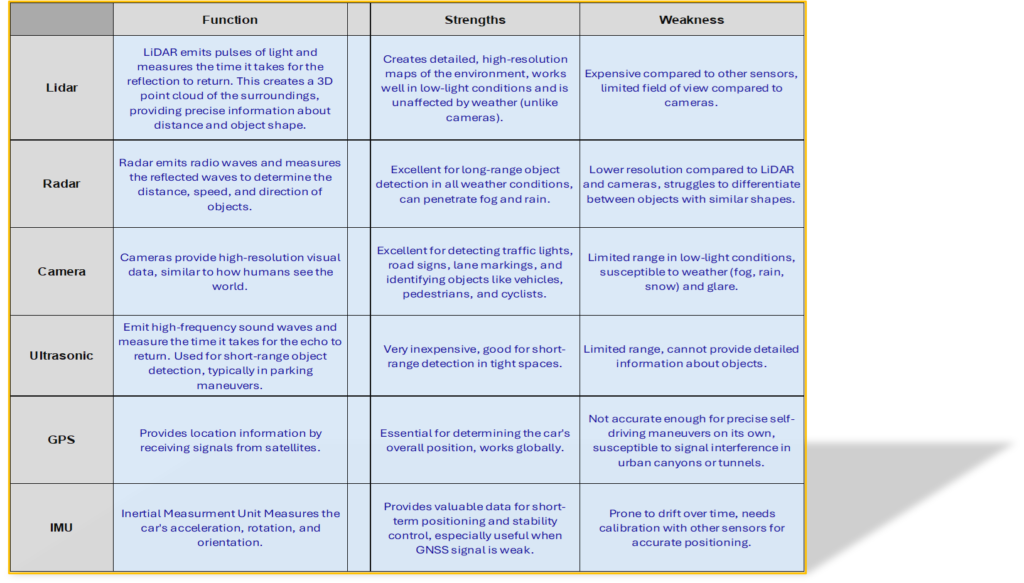
Sensors
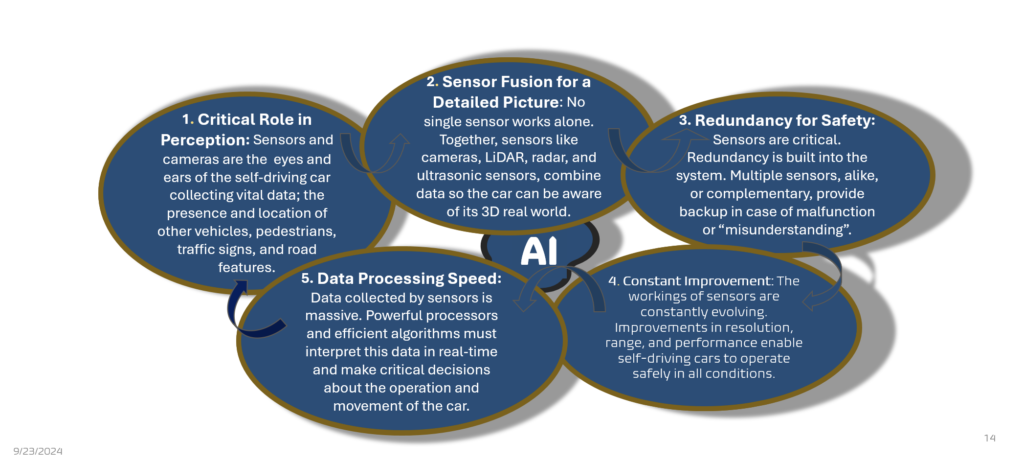
Lidar
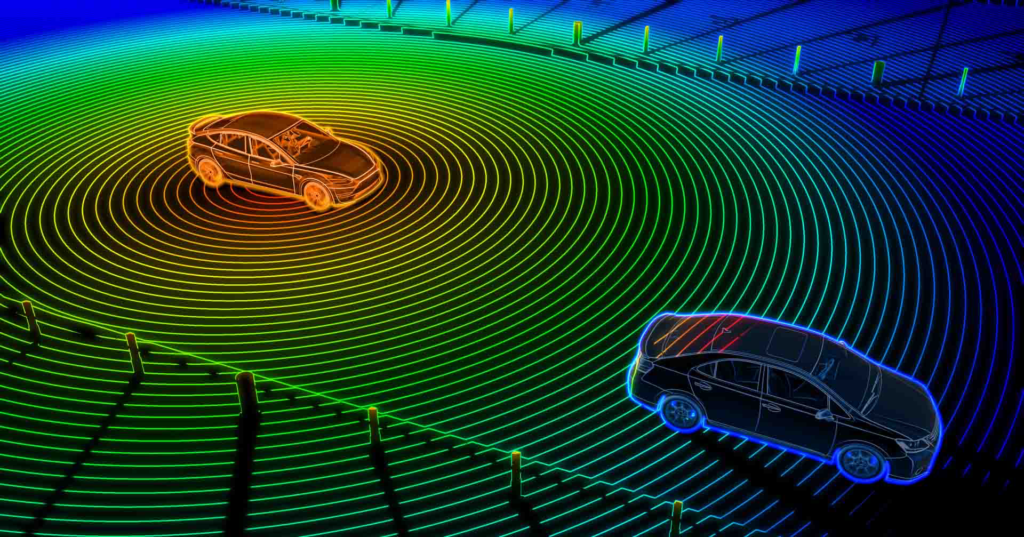
- Technology and Functionality: LiDAR (Light Detection and Ranging)
uses laser pulses to measure distances by calculating the time it takes
for each pulse to bounce back after hitting an object. This data is used
to create precise, high-resolution 3D maps of the surrounding environment. - Applications: LiDAR is widely used in various fields, including autonomous vehicles for navigation and obstacle detection, topographic mapping, forestry management, urban planning, archaeology, and environmental monitoring.
- Components: A typical LiDAR system consists of a laser, a scanner, and a specialized GPS receiver. The laser emits the pulses, the scanner directs the laser across the target area, and the GPS receiver provides location data to create accurate maps.
- Advantages: LiDAR offers high accuracy and resolution, the ability to penetrate vegetation and other obstacles (useful in forestry and environmental studies), and rapid data acquisition over large areas. It’s effective in both day and night conditions, making it versatile for various applications.
- Challenges: LiDAR systems can be expensive and require significant processing power to handle the large volumes of data generated. They can also be affected by adverse weather conditions like heavy rain or fog, which can scatter the laser pulses and reduce accuracy.
Radar

- Detection and Ranging: Radar (Radio Detection and Ranging) uses radio waves to detect objects and measure their distance, speed, and direction. It is crucial for identifying other vehicles, pedestrians, and obstacles in a self-driving car’s surrounding environment.
- Weather Resilience: Unlike other sensors such as cameras and LiDAR, radar performs well in various weather conditions, including rain, fog, and snow. This makes it a reliable sensor for autonomous vehicles in challenging weather.
- Doppler Effect Utilization: Radar systems in self-driving cars use the Doppler effect to measure the relative speed of objects. This helps the car understand how fast other vehicles or pedestrians are moving, which is essential for making safe driving decisions.
- Long-Range Detection: Radar can detect objects at greater distances compared to some other sensor technologies. This long-range capability allows autonomous vehicles to anticipate and respond to potential hazards well in advance, enhancing safety.
- Integration with Other Sensors: Radar is often used in conjunction with other sensors such as cameras and LiDAR in a sensor fusion approach. This integration provides a more comprehensive understanding of the environment, combining the strengths of each sensor to improve the overall reliability and accuracy of the self-driving system.
Ultrasonic
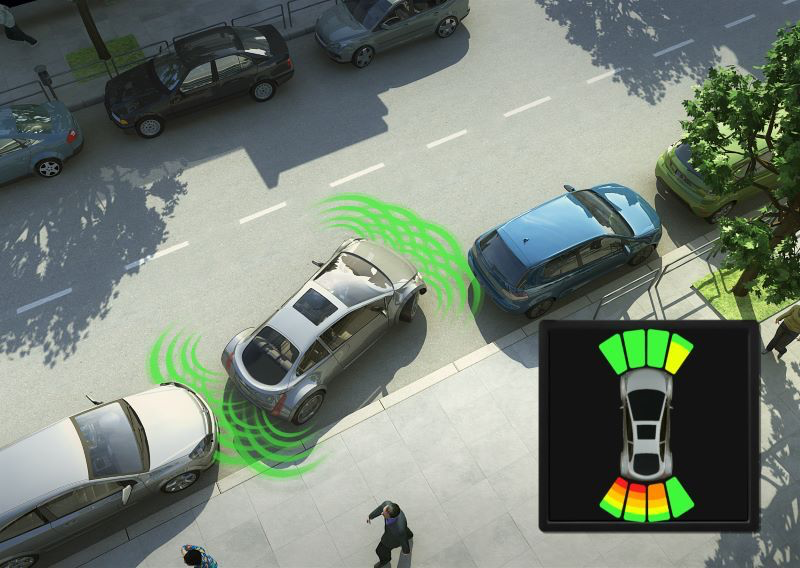
Tesla Fact!
Tesla’s used to come with ultrasonic sensors. To reduce costs Elon Musk decided to switch to a camera based system with already existing cameras on Tesla’s.
- Short-Range Detection: Ultrasonic sensors are highly effective for short-range detection, typically up to a few meters. They are commonly used for parking assistance, detecting obstacles, and low-speed maneuvering in tight spaces.
- Object Proximity Measurement: These sensors measure the distance to objects by emitting ultrasonic waves and calculating the time it takes for the waves to bounce back after hitting an object. This helps self-driving cars understand the proximity of nearby objects.
- Weather and Lighting Independence: Ultrasonic sensors are not affected by lighting conditions, making them reliable for operation in both day and night. They are also relatively unaffected by weather conditions such as rain and fog, which can impair other sensors.
- Cost-Effective: Ultrasonic sensors are relatively inexpensive and easy to integrate into vehicles. This makes them a cost-effective solution for enhancing the situational awareness of self-driving cars, particularly for low-speed applications.
- Integration with Other Systems: Ultrasonic sensors are often integrated with other sensor systems, such as cameras and radar, to provide a comprehensive understanding of the vehicle’s surroundings. This integration helps improve the accuracy and reliability of obstacle detection and avoidance, particularly in complex environments like parking lots.
Camera
- Image Recognition: Cameras provide high-resolution images and video feeds that are essential for image recognition and computer vision algorithms. They enable self-driving cars to recognize and classify objects such as traffic signs, pedestrians, lane markings, and other vehicles.
- Color and Texture Information: Unlike other sensors like LiDAR and radar, cameras capture detailed color and texture information. This is crucial for tasks such as traffic light recognition, reading road signs, and identifying lane markings.
- Affordability and Ubiquity: Cameras are relatively inexpensive and widely available compared to other sensor technologies. This makes them an attractive option for equipping autonomous vehicles with the necessary sensing capabilities without significantly driving up costs.
- Close-Range Detection: Cameras excel at detecting objects at close range and providing detailed visual information. This is particularly useful for tasks such as parking, navigating through complex urban environments, and avoiding obstacles.
- Complementary Sensor Role: In a self-driving car’s sensor suite, cameras complement other sensors like LiDAR and radar. By integrating data from multiple sensors (sensor fusion), cameras help provide a more complete and accurate understanding of the vehicle’s surroundings, enhancing overall safety and performance.
Software and Algorithms
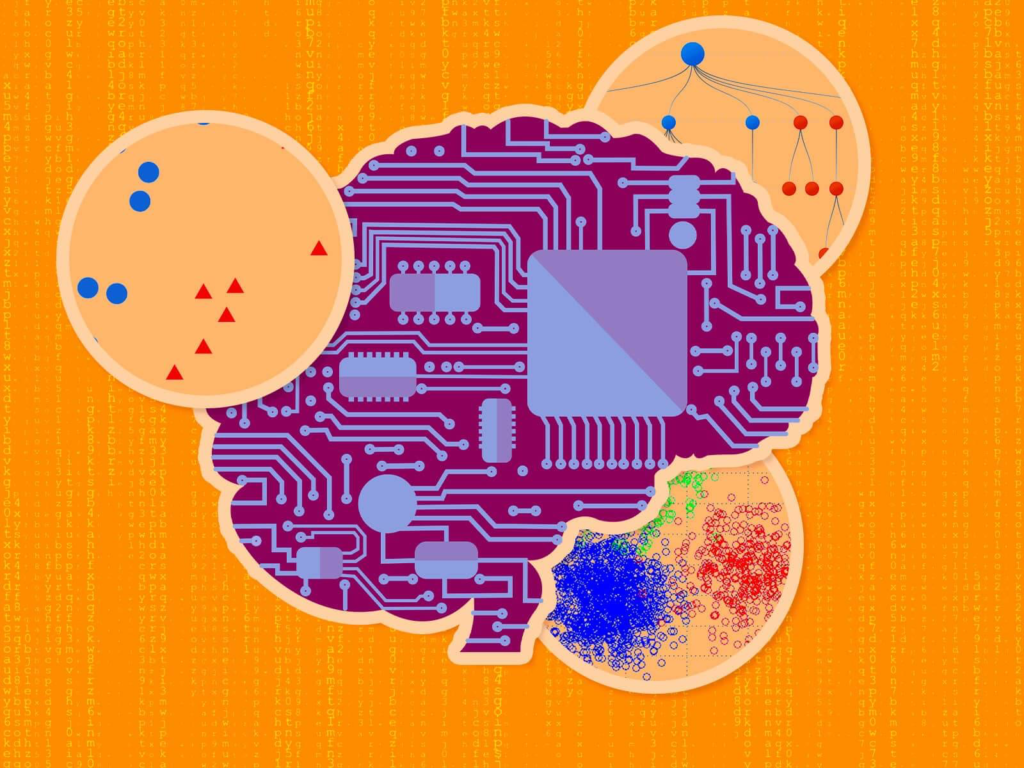
Perception and localization are fundamental components in autonomous driving systems, enabling the vehicle to understand its surroundings and position. Perception processes data from various sensors, such as cameras and LiDAR, to detect objects, pedestrians, traffic signs, and road markings. Machine learning and computer vision techniques are employed to classify these elements in real time. Localization, on the other hand, determines the vehicle’s precise location using GPS, inertial measurement units (IMUs), and sensor data. This real-time data is combined with pre-mapped information to provide an accurate understanding of the vehicle’s current position within its environment.
Once the vehicle has a clear sense of its surroundings and location, planning and decision-making algorithms create a safe path to follow. These systems consider the vehicle’s destination and environmental factors, such as nearby objects and traffic, to make real-time decisions like changing lanes or stopping for pedestrians. Control systems then execute these plans by managing steering, acceleration, and braking to ensure smooth movement, adapting to road conditions and the vehicle’s speed. Connectivity further enhances these processes by enabling communication with other vehicles, infrastructure, and pedestrians, sharing real-time data about road conditions. Cloud computing also plays a crucial role, providing additional computational power, enabling software updates, and enhancing the vehicle’s AI-driven decision-making through real-world data and simulations. AI and machine learning systems are integral in refining perception, planning, and control by continuously learning from real-world and simulated experiences.
Human-Machine Interface
In the world of self-driving cars, the Human-Machine Interface (HMI) acts as a bridge between the automated driving system and the human occupants. It’s essentially a communication system that allows the car to:
- Inform the driver: The HMI keeps the driver informed about the car’s status, upcoming maneuvers, potential hazards, and the overall driving environment. This might involve visual displays on dashboards or heads-up displays (HUDs), as well as audible alerts or voice messages.
- Receive driver input: While a self-driving car aims to handle most situations autonomously, there might be scenarios where human intervention is necessary. The HMI provides a way for the driver to take control of the vehicle, disengage self-driving features, or input commands if needed. This could involve buttons on the steering wheel, touchscreens, or voice commands.
Some key characteristics of a well-designed HMI for self-driving cars:
- Clarity and Simplicity: The information presented through the HMI should be clear, concise, and easy for the driver to understand. Avoidance of technical jargon and use of intuitive visuals are important.
- Minimal Distraction: The HMI shouldn’t overload the driver
with unnecessary information or complex interfaces. It
should be designed to minimize distraction while keeping
the driver informed of critical situations. - Timely and Accurate Information: The information displayed through the HMI needs to be timely and accurate to allow the driver to make informed decisions when necessary.
- Trust and Transparency: A good HMI fosters trust between the driver and the self-driving system. It should provide clear indications of the car’s capabilities and limitations, and when human intervention might be required.
- Adaptability: The HMI should ideally be adaptable to different driving situations and user preferences. For example, it might display more information when the car is in manual mode compared to fully autonomous driving.
Since self-driving car technology is still evolving, HMI design is an important area of research and development. The goal is to create a seamless and intuitive interface that fosters trust, keeps the driver informed, and ensures a safe and comfortable driving experience.
Major Players
Waymo vs. Tesla Debate
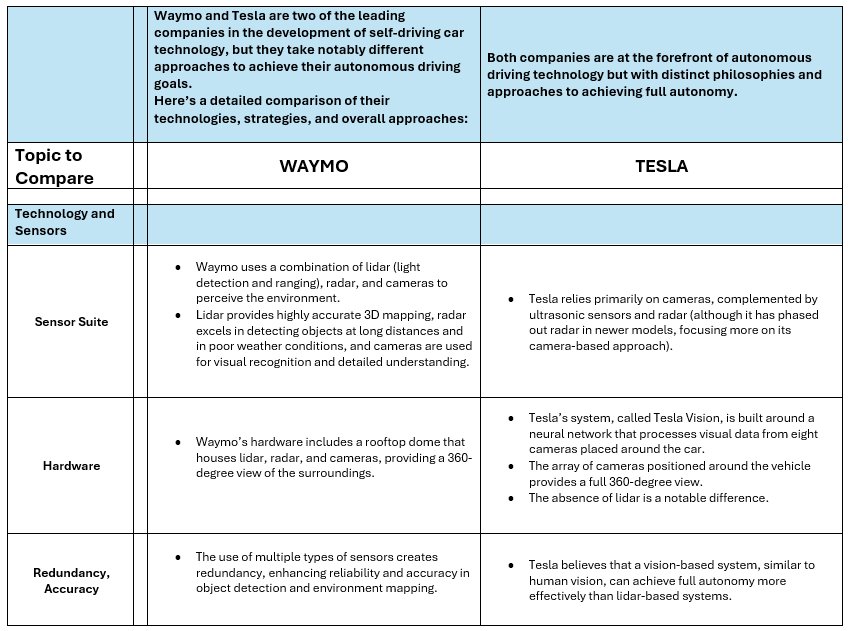
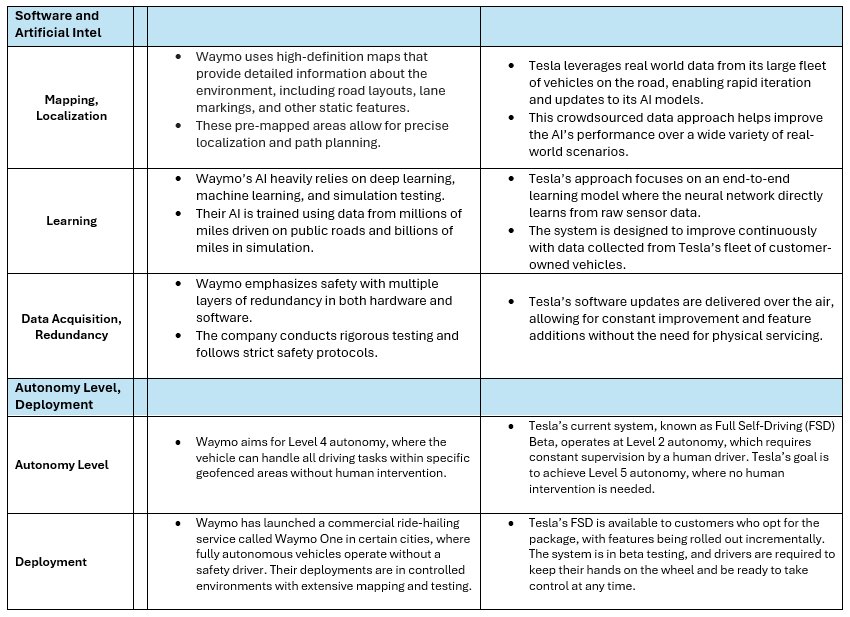
Others
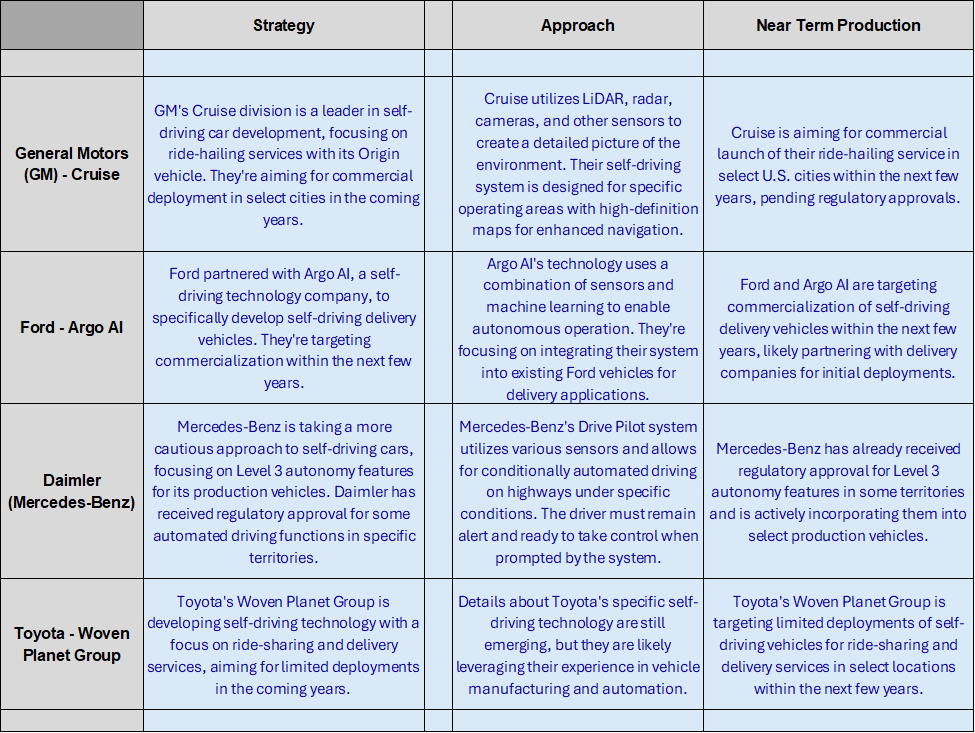
Ethical Considerations & Hurdles
As the use of autonomous vehicles (AVs) becomes more common, there is a complex web of legal and policy challenges intertwined with ethical considerations. No doubt some of these are yet to even be imagined. Here are some of the more apparent problems.
Liability and Responsibility:
- Accidents: In an accident involving an AV, who is liable? The manufacturer, programmer, or owner? Current laws are designed for human drivers, and assigning blame to non-human entities needs legal frameworks.
- Transparency: How accidents involving AVs are investigated is crucial. Understanding the decision-making process of the car’s AI is essential for assigning blame and improving future safety.
Ethical Programming:
- Moral Dilemmas: Self-driving cars will face situations where an accident is unavoidable (trolley problem). How should the car’s AI be programmed to handle these situations? Who takes priority (passengers, pedestrians, etc.)? Policymakers need to establish ethical guidelines for programming AV decision-making.
- Data Bias: The algorithms powering AVs are trained on vast amounts of data. If this data is biased, the car’s decision-making could be unfair or discriminatory. Regulations are needed to ensure unbiased data collection and training of AV AI.
Privacy Concerns:
- Data Collection: Self-driving cars gather a lot of data about their surroundings and passenger journeys. Data privacy laws need to be adapted to address the collection, storage, and use of this data.
- Cybersecurity: AVs are vulnerable to hacking. Policymakers need to establish cybersecurity standards to protect AV systems from malicious attacks.
Social and Regulatory Issues:
- Accessibility: Ensuring equitable access to AV technology is important. Regulations should address potential discrimination based on factors like income or disability.
- Job Displacement: The rise in AVs might lead to job losses in the transportation sector (taxi drivers, truck drivers). Policymakers need to consider retraining programs and social safety nets to address these challenges.
These are just some of the legal and policy implications arising from the ethical considerations surrounding AVs. Addressing these issues is crucial for building public trust and ensuring the safe and responsible deployment of autonomous vehicles.
International Cooperation:
Due to the global nature of transportation, international cooperation in developing legal and policy frameworks for AVs is crucial. This will help ensure consistent safety standards and ethical considerations across borders.
What the Future Holds
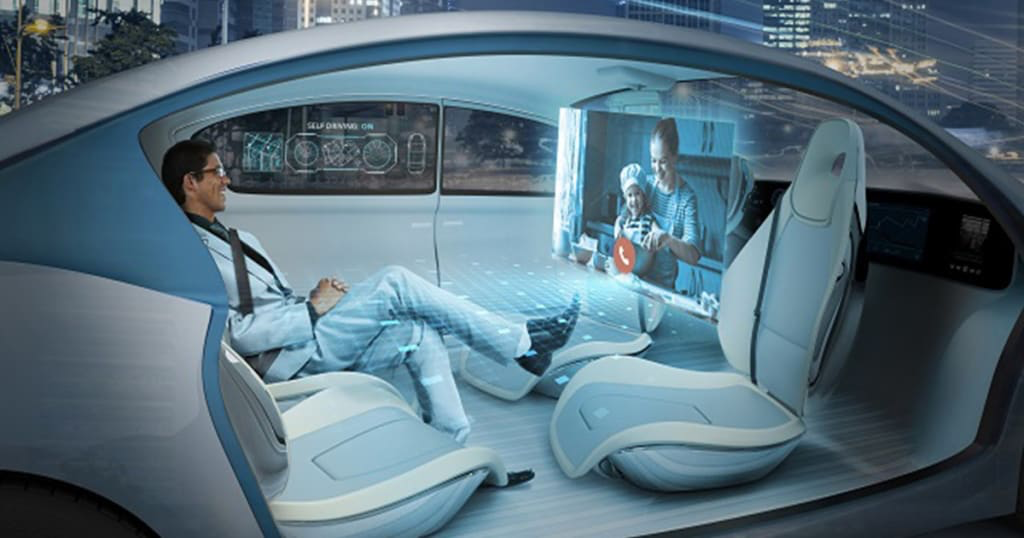
Self-driving cars are on the horizon, promising a revolution in transportation. Advancements in machine learning and a shift in strategy by key players paint a picture of a future with exciting possibilities with problems to resolve.
The Engine of Progress: Machine Learning
Machine learning is the engine powering self-driving cars. These algorithms ingest massive amounts of data to “see” the world through cameras and sensors, recognize objects, and make decisions like maintaining a safe following distance or navigating an intersection. As machine learning continues to evolve, so will the capabilities of self-driving cars.
- A Phased Approach – Early adopters, gradual expansion, integration with smart infrastructure
- Reshaping Transportation – Ride-hailing revolution, shifting ownership or subscription models, complements public transportation, seamless network.
- A World Transformed – Safer roads, increased efficiency, accessibility for all
- Cities Reimagined – Reclaimed streets, smarter Infrastructure, evolving urban design: slower speeds, more people-friendly
Challenges on the Road Ahead
While the future holds promise, there are hurdles to overcome:
- Ethical Dilemmas: Who is responsible in case of an accident with a self-driving car? How should these vehicles be programmed to handle unavoidable situations?
- Job Displacement: The rise of self-driving cars could lead to job losses in the transportation sector. Retraining programs and social safety nets will be crucial.
- Privacy Concerns: The vast amount of data collected by self-driving cars raises privacy concerns. Robust data security measures will be essential.
Collaboration is Key
The future of self-driving cars hinges on collaboration between various stakeholders:
- Tech Companies: Continued investment in R&D
- Policymakers: Clear regulations, safety standards for acceptance
- Urban Planners: adapt infrastructure, accommodation
A Future Worth Driving Towards
By addressing the challenges and embracing the opportunities, self-driving cars have the potential to create a safer, more efficient, and equitable transportation system for everyone. The road ahead might have its bumps, but the destination – a world transformed by self-driving cars – promises to be an exciting one.
Tesla’s Robotaxi Event: We Robot
Video by: Auto Focus Youtube Channel.
Tesla’s highly anticipated “We, Robot” event on October 10, 2024, revealed their latest innovation: the Cybercab, a fully autonomous robotaxi. The event, held at Warner Bros. Studios in Los Angeles, showcased Tesla’s vision for a future dominated by driverless, electric vehicles. Elon Musk emphasized how these vehicles will transform urban mobility by offering affordable, on-demand transportation that eliminates the need for human drivers.
Key highlights from the event:
- Cybercab Design: Drawing inspiration from Tesla’s Cybertruck, the Cybercab robotaxi is built for autonomy, lacking a steering wheel or pedals. Musk announced that it will be available for under $30,000, offering consumers the option to purchase it for personal use, though the primary goal is to build a fleet for public robotaxi services.
- Autonomy and Safety: Tesla plans to move from “supervised” Full Self-Driving (FSD) to unsupervised FSD, where vehicles will operate entirely without human oversight. Musk claimed that these robotaxis would be ten times safer than human drivers, with AI and sensors constantly vigilant and not subject to distractions.
- Economic and Environmental Benefits: With robotaxis, Tesla envisions a reduction in private car ownership, making point-to-point transportation more efficient and reducing urban congestion. This change could lead to the reclamation of parking spaces for parks or other community uses.
Tesla’s Cybercab robotaxi marks a significant step toward affordable, autonomous transport, which could revolutionize both the ride-hailing industry and urban planning in the near future.
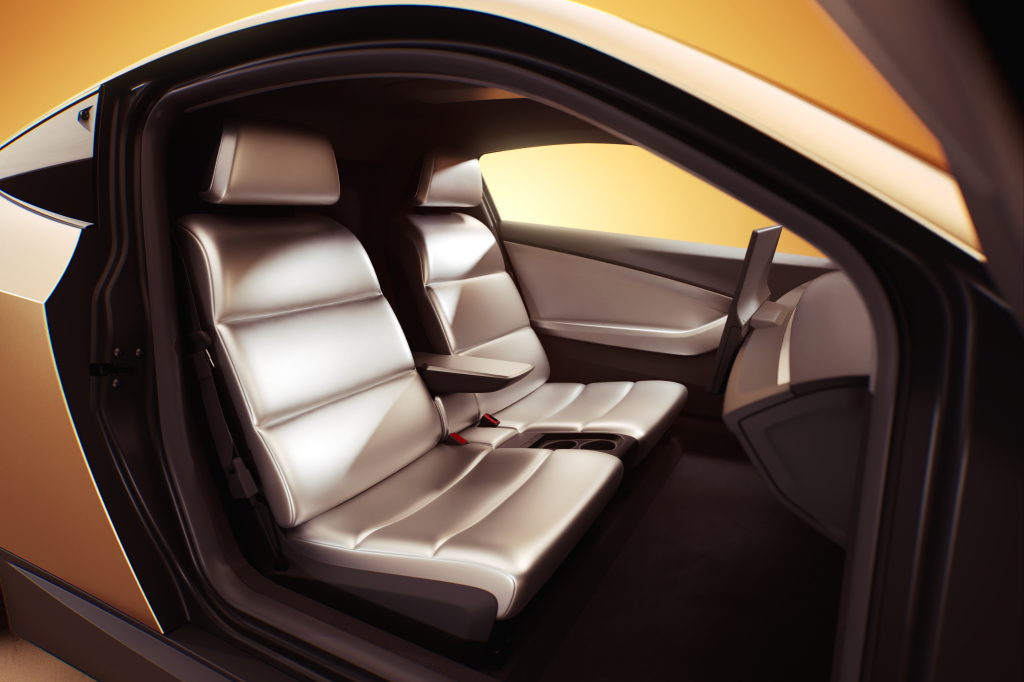
Conclusions
A Revolution on the Road
- Autonomous vehicles (AVs), also known as self-driving cars, are the future of transportation, promising a world where cars navigate and operate without human input. Here are main points about their emergence, technology, impact, and potential future:
The Rise of the Machines
The concept of self-propelled vehicles dates back centuries, but modern AVs emerged from advancements in various fields. Early iterations involved automating simple tasks like cruise control (1950s). Today’s AVs rely on a complex suite of technologies including:
- Sensors: LiDAR, radar, and cameras capture a 360-degree perception of the environment.
- Algorithms: Complex software interprets sensory data to identify objects, predict movements, and make driving decisions.
- Machine Learning: AVs continuously learn and adapt by processing massive amounts of driving data.
The Road Ahead
Despite the challenges, the emergence of AVs is an unstoppable force. Predictions on the exact timeline vary, but experts suggest a significant presence of AVs on roads by 2030. Here are some possible future scenarios:
Phased Adoption: A gradual integration of AVs, with initial use in controlled environments like highways or ride-sharing services.
Transformative Impact: A complete overhaul of transportation systems, with personal car ownership declining and AV mobility becoming the norm.
The future of AVs hinges on technological advancements, effective regulation, and public acceptance. But one thing is certain: autonomous vehicles are poised to revolutionize how we travel, potentially creating a safer, more efficient, and accessible transportation landscape.
Hands on Activities
Activities provide opportunities to engage grade 7-12 students in hands-on learning experiences, exploring aspects of artificial intelligence and self-driving cars. Through critical thinking exercises, and collaborative projects participants deepen their understanding beyond the classroom setting, fostering creativity and problem-solving skills.
Step 1: Robotics Workshop – Build a Mini Self-Driving Car
Organize a robotics workshop where students build small-scale autonomous vehicles (robots). Provide students with kits or materials to build small-scale self-driving car prototypes. Examples of kits include LEGO Mindstorms, mBot, or Arduino-based platforms.
- Appendix A Contains a workbook for the basic mBot Educational Robot Kit. The workbook will show students how to get started, unboxing the kit, understanding its contents, building the mBot car, and operating mBot for the first time, after building it.
- Guide students through assembling the car chassis, attaching sensors (such as ultrasonic sensors or infrared sensors), and understanding the functions of the remote control keypad, and the built-in movements of mBot.
- Guide students in programming these robots to navigate a predefined course, simulating the challenges faced by self-driving cars.
- Encourage students to experiment with different sensor configurations and algorithm parameters to improve their car’s performance.
Step 2: Data Analysis and Visualization
- Provide students with real-world data sets related to traffic patterns, accident statistics, or environmental conditions.
- Have students analyze the data to identify trends and patterns that could inform AI algorithms in self-driving cars, emphasizing the importance of data-driven decision-making.
- Provide students with datasets containing sensor data collected from real or simulated self-driving car scenarios.
- Guide students through the process of analyzing the data, identifying patterns, and visualizing the results using tools like Microsoft Excel, Google Sheets, or data visualization libraries in Python.
- Encourage students to interpret their findings and draw insights about factors influencing autonomous driving performance, such as weather conditions or traffic density.
There are a few resources you can explore to find datasets on automobile traffic scenarios, accident statistics, and environmental conditions. Here are a couple of options:
- National Highway Traffic Safety Administration (NHTSA) Data: The NHTSA publishes a wealth of data related to traffic safety in the United States. They offer datasets on various topics including traffic crashes, fatalities, injuries, and contributing factors [https://www.nhtsa.gov/](https://www.nhtsa.gov/). You might need to search within the website to find datasets that specifically link crash data with environmental conditions.
- Open Government Data: Many government agencies around the world publish open data sets. You can search for data portals of specific countries, states, or cities you’re interested in. Look for datasets related to traffic collisions, weather conditions, and road infrastructure.
- Kaggle: Kaggle is a platform for data science where researchers and organizations share datasets. You can search for datasets related to traffic accidents and environmental factors. Keep in mind that the quality and format of datasets on Kaggle can vary.
While searching for datasets, consider filtering by factors like location (country, city), timeframe, and specific crash types (e.g., single-vehicle, multiple-vehicle) to find data most relevant to your needs. It’s important to note that finding a dataset that directly combines accident statistics with environmental conditions might require some data manipulation or merging information from separate datasets.
Step 3. Ethical (Dilemma) Debate or Panel Discussions
- Present students with hypothetical scenarios involving ethical dilemmas faced by self-driving cars, such as deciding between protecting passengers or pedestrians in emergency situations.
- Facilitate group discussions where students debate the ethical considerations and implications of different decision-making approaches.
- Encourage students to articulate their reasoning and consider the broader societal impacts of autonomous vehicle ethics.
- Organize a class debate or panel discussion on ethical issues related to AI and self-driving cars.
- Assign students roles representing different stakeholders (e.g., engineers, legislators, consumers) to discuss topics like safety, privacy, and societal impact.
No Fields Found.
Copyright 2024 Mascatello Arts, LLC All Rights Reserved
SteamPilots.com
Mascatelloarts.com